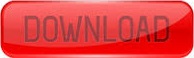
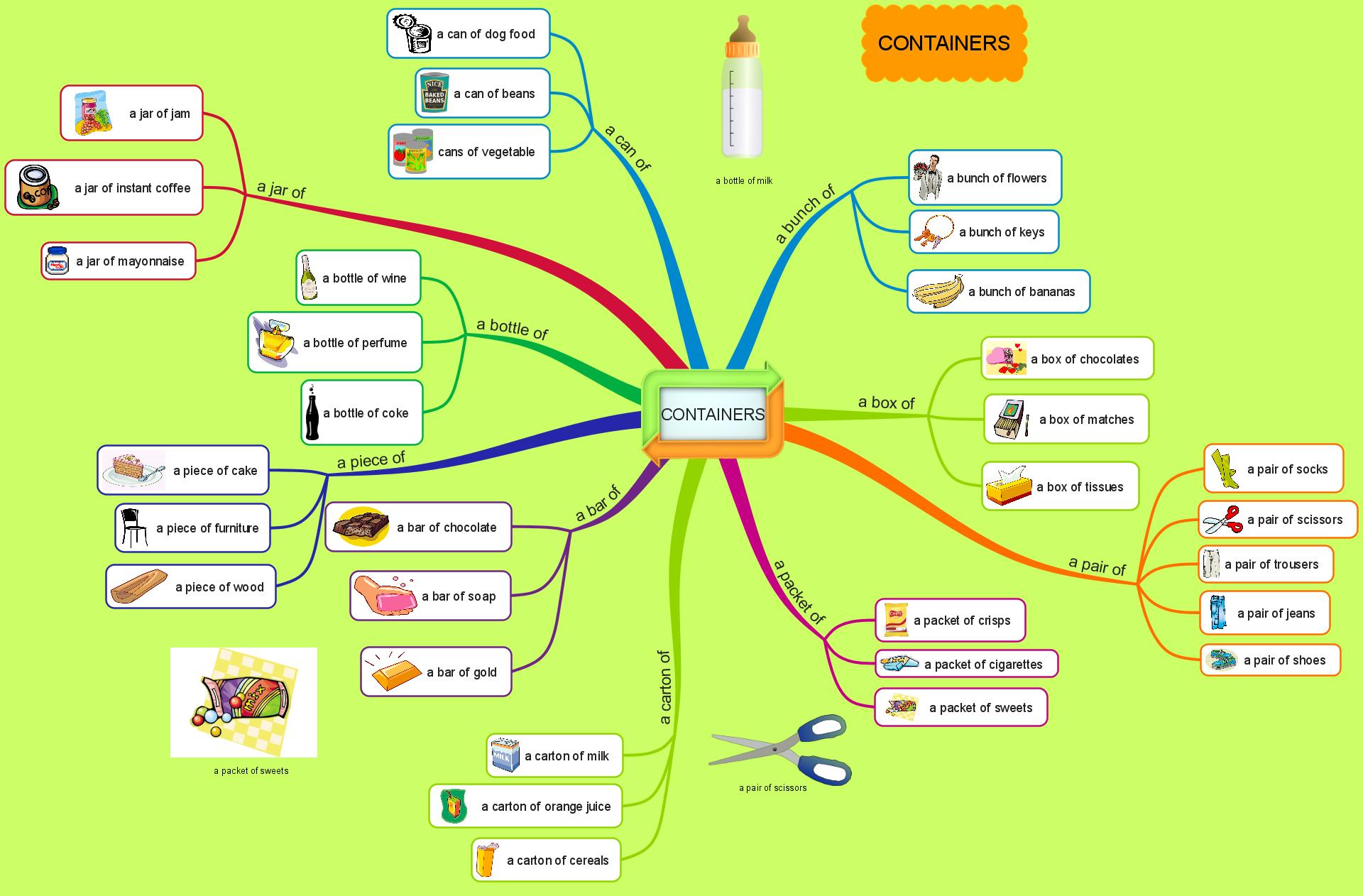
Treenity mind map Offline#
D-Lib Magazine - The Magazine of Digital Library Research 20(11/12) (2014)īeel, J., Langer, S.: A Comparison of Offline Evaluations, Online Evaluations, and User Studies in the Context of Research-Paper Recommender Systems. Springer, Heidelberg (2014)īeel, J., Langer, S., Gipp, B., Nürnberger, A.: The Architecture and Datasets of Docear’s Research Paper Recommender System. In: de Rijke, M., Kenter, T., de Vries, A.P., Zhai, C., de Jong, F., Radinsky, K., Hofmann, K. Hofmann, K., Schuth, A., Bellogın, A., de Rijke, M.: Effects of Position Bias on Click-Based Recommender Evaluation. Program: Electronic Library and Information Systems 47(1), 92–112 (2013)īuzan, T.: The Mind Map Book (Mind Set). Zarrinkalam, F., Kahani, M.: SemCiR - A citation recommendation system based on a novel semantic distance measure. Göksedef, M., Gündüz-Ögüdücü, S.: Combination of Web page recommender systems.
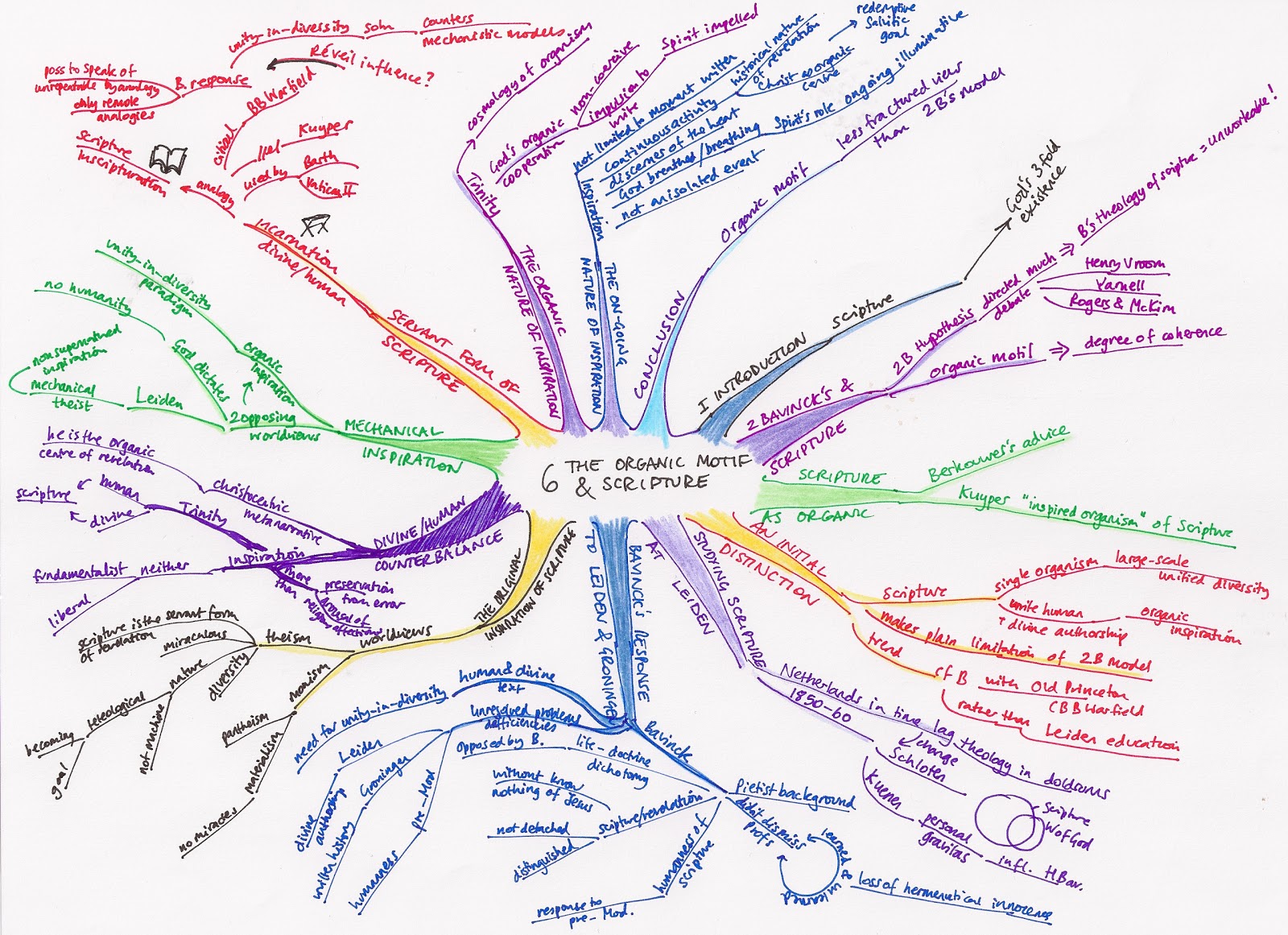
In: Bui, L.T., Ong, Y.S., Hoai, N.X., Ishibuchi, H., Suganthan, P.N. Ha, Q.M., Tran, Q.A., Luyen, T.T.: Personalized Email Recommender System Based on User Actions. In: Lalmas, M., Jose, J., Rauber, A., Sebastiani, F., Frommholz, I. 123–127 (2012)īia, A., Muñoz, R., Gómez, J.: Using Mind Maps to Model Semistructured Documents. In: Proceedings of the 2nd IEEE International Conference on Computer Science and Automation Engineering (CSAE), pp.
Treenity mind map generator#
Kudelic, R., Malekovic, M., Lovrencic, A.: Mind map generator software.
Treenity mind map software#
Holland, B., Holland, L., Davies, J.: An investigation into the concept of mind mapping and the use of mind mapping software to support and improve student academic performance (2004) Contributions on Information Systems and Technologies, 1 (2013) H.E., Palácios, C.G., LErario, A., Domingues, A.L., Gonçalves, J.A., Duarte, A.S., Fabri, J.A.: Applying Mind Maps at Representing Software Requirements. Sixth International Conference on Advanced Learning Technologies, pp. Zualkernan, I.A., AbuJayyab, M.A., Ghanam, Y.A.: An alignment equation for using mind maps to filter learning queries from Google. In: International Conference on Computer and Electrical Engineering, pp. Springer, Heidelberg (2014)Ĭhien, L.-R., Buehre, D.J.: A Visual Lambda-Calculator Using Typed Mind-Maps. In: Dimitrova, V., Kuflik, T., Chin, D., Ricci, F., Dolog, P., Houben, G.-J. Keywordsīeel, J., Langer, S., Genzmehr, M., Gipp, B.: Utilizing Mind-Maps for Information Retrieval and User Modelling. Our research shows that mind map-specific user modeling has a high potential, and we hope that our results initiate a discussion that encourages researchers to pursue research in this field and developers to integrate recommender systems into their mind mapping tools. A user study confirmed the high effectiveness of the mind map specific approach with an average rating of 3.23 (out of 5), compared to a rating of 2.53 for the best baseline. However, when adjusting user modeling to the unique characteristics of mind maps, a higher CTR of 7.20% could be achieved. The evaluation shows that standard user modeling approaches are reasonably effective when applied to mind maps, with click-through rates (CTR) between 1.16% and 3.92%. Docear displayed 430,893 research paper recommendations, based on 4,700 user mind maps, from March 2013 to August 2014.
:max_bytes(150000):strip_icc()/Screen-shot-2013-10-29-at-10.07.35-AM-56a2aefa5f9b58b7d0cd607e.png)
The approaches are applied and evaluated using our mind mapping and reference-management software Docear. Additionally, we develop novel user modeling approaches that consider the unique characteristics of mind maps. In this paper, we explored the effectiveness of standard user-modeling approaches applied to mind maps. Mind maps have not received much attention in the user modeling and recommender system community, although mind maps contain rich information that could be valuable for user-modeling and recommender systems.
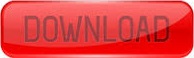